The International Institute for Analytics (IIA) has released its list of predictions for analytics trends in 2016. Unlike in years past, the IIA is dividing its list into two distinct groups -- the first focused on analytics predictions; the second focused on analytics priorities. IIA co-founder Tom Davenport, and researchers Dan Magestro and Robert Morison, discussed the predictions and priorities during a recent webinar.
Plus, predicting analytics trends year after year can be repetitive. "The hot topics take a while to implement, and they take a while to come to fruition," said Davenport, the president's distinguished professor of information technology and management at Babson College in Babson Park, Mass., and an analytics thought leader."In the discussions we've had with leaders and practitioners across enterprise organizations and across industries, we've heard a lot of consistent themes, and pressing, urgent needs. And we thought this would be a great chance to provide a concise view of the priorities and themes we're hearing about," said Magestro, a former analytics practitioner at Cardinal Health, JPMorgan Chase & Co. and Nationwide Insurance, who now leads research efforts at the IIA.
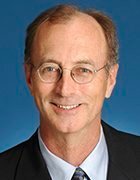
The Portland, Ore., advisory and research organization's list of five analytics trends includes a mix of advanced technologies and predictions that suggest analytics will become more of a generic, everyday thing in 2016. "And having analytics as more of an everyday thing is a reflection of advancing maturity," said Morison, analytics consultant and researcher at the IIA and webinar moderator.
The IIA's five analytics predictions are as follows:
No. 1: Cognitive technology subsumes automated analytics
CIOs probably see automated analytics, which takes action on the results of its analyses, as different from cognitive technology, commonly associated with technologies such as IBM Watson. But in 2016, the distinction between the two will begin to fade, according to Davenport.
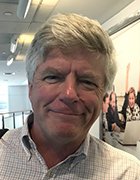
The line separating the two is already "relatively thin," he said, since many of the technologies underlying cognitive computing -- such as logistic regression, deep learning and neural networks -- are already well-known to analytics professionals. "Cognitive technologies are a way to repackage them along with a lot of other capabilities organizations need these days in order to ease knowledge bottlenecks and to deal with more information than humans can really comprehend," Davenport said.
The prediction could be good news for CIOs and analytics professionals alike. While data has taken a more prominent role in just about every organization, managers still tend to rely on criteria other than analyses to make decisions. But integrating tools that take on some decision-making functions automatically will mean analytics can't be avoided, Davenport said.
No. 2: Analytical microservices embedded into processes
Traditional analytics is often thought of as a package of capabilities, but increasingly, one specific analytical technique -- an analytical microservice -- is embedded into systems and processes, often through APIs. Davenport sees the analytics trend continuing in 2016.
"Those of you who follow IBM Watson know that it's no longer a monolithic Q&A application," he said. Watson can now provide 32 different APIs, including text analysis, text translation and scoring.
An interesting side note: Early adopters of IBM Watson technology have often been domain specialists, such as surgeons or oncologists, especially since healthcare was a primary focus for the technology, according to Davenport. "But as this becomes an enterprise-wide focus, and we do more of these kinds of applications, I think it will, in most cases, be combined with the analytics groups within companies," he said.
No. 3: Data science, predictive and prescriptive analytics merge
Big data talk early on made it sound like CIOs and analytics practitioners had to think of it as something wholly separate from traditional analytics, even requiring a distinct group to corral and mine the swaths of data coming at businesses. According to IIA research, that perspective is changing. "These things are, in many cases, being combined, and data science, if anything, is becoming a specialized branch of the central analytics group," Davenport said.
In 2016, the distinction between data science and analytics will continue to blur and become muddled, as big data gets more deeply integrated into more traditional businesses. "We'll see entire analytics groups being called data science groups and vice versa," he said.
No. 4: The analytics talent crunch eases
CIOs probably know this 2011 McKinsey prediction by heart: By 2018, the United States will experience a shortage of 140,000 to 190,000 skilled data scientists. But, according to Davenport, the pains of finding good analytics talent have subsided -- to a degree.
Businesses are taking advantage of university programs that are turning out specialized analytics talent, or they've created "retread programs" to train analytics employees in data science or business employees in analytics, he said. He pointed to Cisco as one such example, which has not only focused on strengthening in-house data science talent, "but is also creating executives who understand how this works, and can pull it into their business units and functions," he said.
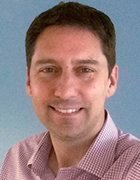
Not everyone agrees with Davenport. "It really depends on who you are, where you are, what industry you're in and if there are interesting challenges," IIA's Magestro said. He pointed to two Midwestern companies, which he wouldn't name, that spoke to him about the difficulty of convincing talent to move to their cities. "The crunch maybe in aggregate isn't as acute as had been forecasted, but at a more micro-level and at the regional level, you can see some of this," he said.
No. 5: Analytics now curates, manages data
Data preparation is one of the biggest time sinks for analytics professionals and data scientists. But new tools are emerging that apply "the analytics we use typically to analyze data to curate data," Davenport said. Rather than approach data management from a centralized, top-down approach, tools from the likes of Tamr -- where Davenport is an advisor -- Paxata andTrifacta work from the bottom up, leveraging machine learning to curate and clean the data. Davenport said the tools have blossomed in the last year or so and will continue to do so in 2016.
"Things are just too complex to do this from the top down at a large organization," Davenport said. "It's not uncommon to have 5,000 different data sources that relate to the same thing in some organizations."
0 comments:
Post a Comment